In my new paper with Georgios Spithourakis and Sebastian Riedel, we use recurrent neural networks to predict clinical codes from patients' discharge summaries. In doing so, we grapple with the idiosyncracies of clinical text and develop a method for learning better representations of rare diseases.
A man attends the emergency department of his local hospital, having developed fevers and breathlessness. Whilst being assessed, he interacts with numerous nurses, doctors and other hospital staff. His vital signs are continuously measured, and he is subjected to a barrage of tests. He is diagnosed with pnuemonia, but deteriorates despite initial therapy and is admitted to the intensive care unit.
Free text and the clinical narrative
In the course of this man's treatment and eventual recovery, his data will be recorded in a variety of administrative, monitoring, laboratory and radiology systems. Amongst all these data, where is the story of this man's illness told?
Due to its high expressiveness and efficiency, free text is the natural choice for a busy clinician trying to quickly record the details of this man's story. However, whilst text notes work well at the point of care, they are often inconvenient for gaining insight into patient populations as a whole. If we are trying to coordinate the care of all the patients into a large hospital, we need a better way of working out what disease each person is being treated for than reading their individual notes.
Getting structured data from text
Enter clinical coding: the task of tagging patients' notes with standardised labels. For example, our patient would end up being assigned the label pneumococcal pneumonia. The labelling systems (ontologies) are usually organised hierarchically:
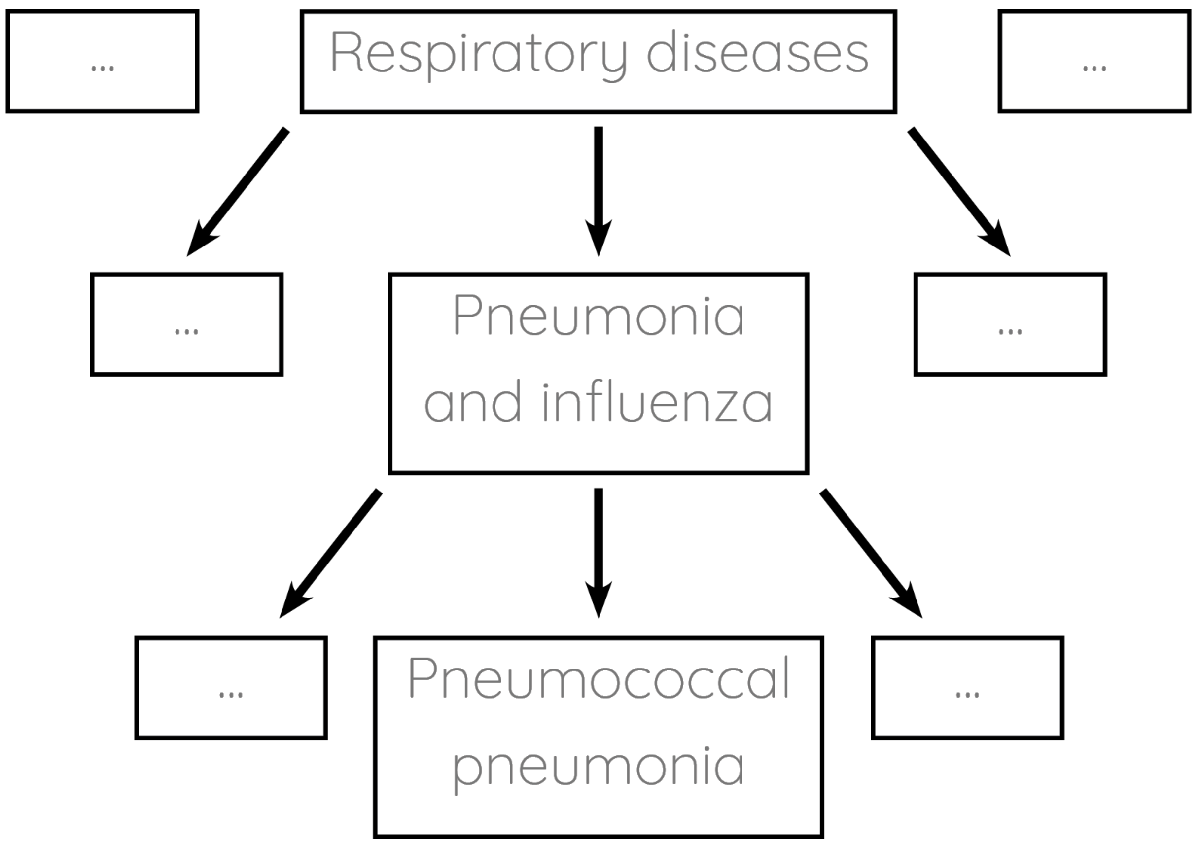
Clinical coding is currently done manually by large teams of hospital staff. Manual coding is an expensive and time-consuming process which typically only gets completed several weeks after patients leave hospital. Numerous studies demonstrate that it is also prone to error. If we could automate clinical coding instead, we could save resources and use the codes for realtime analytics.
Problem 1: Notes are hard to read
The task is clear. We need a system which reads patients' notes and automatically tags them with clinical codes. What might the notes for our patient with pnuemonia look like?
72M presented with 3/7 worsening DIB, cough productve green sputum++, spiking temps, tachy, BP 82/40. CXR - dense LLL consolidation. CRP 274 WCC 23.6 (85% neuts). PE, due to no signs DVT and better alternate dx was felt unlikly. Rx w/ fluids, IV coamox+clari. Transferred to ICU for pressor support. Abx later switched to PO.
Text like this is challenging for machines to read. It is formatted is ad hoc, and is full of spelling mistakes and ambiguous abbreviations. The same concepts are referred to by multiple synonyms, and are sometimes mentioned earlier in the document only to be negated several words later (so you couldn't identify the negation by simple pattern matching). Whilst the diagnosis is heavily implied by the text, it is never mentioned explicitly.
As human readers, we overcome many of these challenges by interpreting each word in the text in the context of the previous words. Our study uses a recurrent neural network (RNN) to mimic this process. We first map the words in the text to word vectors. Because synonyms are used in similar settings, their corresponding points in the word vector space end up close together. We input the word vectors sequentially into the RNN, which updates its internal state in a manner which depends on both the current state and the current input.
A simplified version of the model we use in our study is shown below. \(\mathbf{o_1}\), \(\mathbf{o_2}\), \(\mathbf{o_3}\) and \(\mathbf{o_4}\) are the internal states (these are vectors too) which the RNN outputs after each word input. Once all the word vectors have been fed into the RNN, its final output serves as a context-aware representation of the text as a whole.
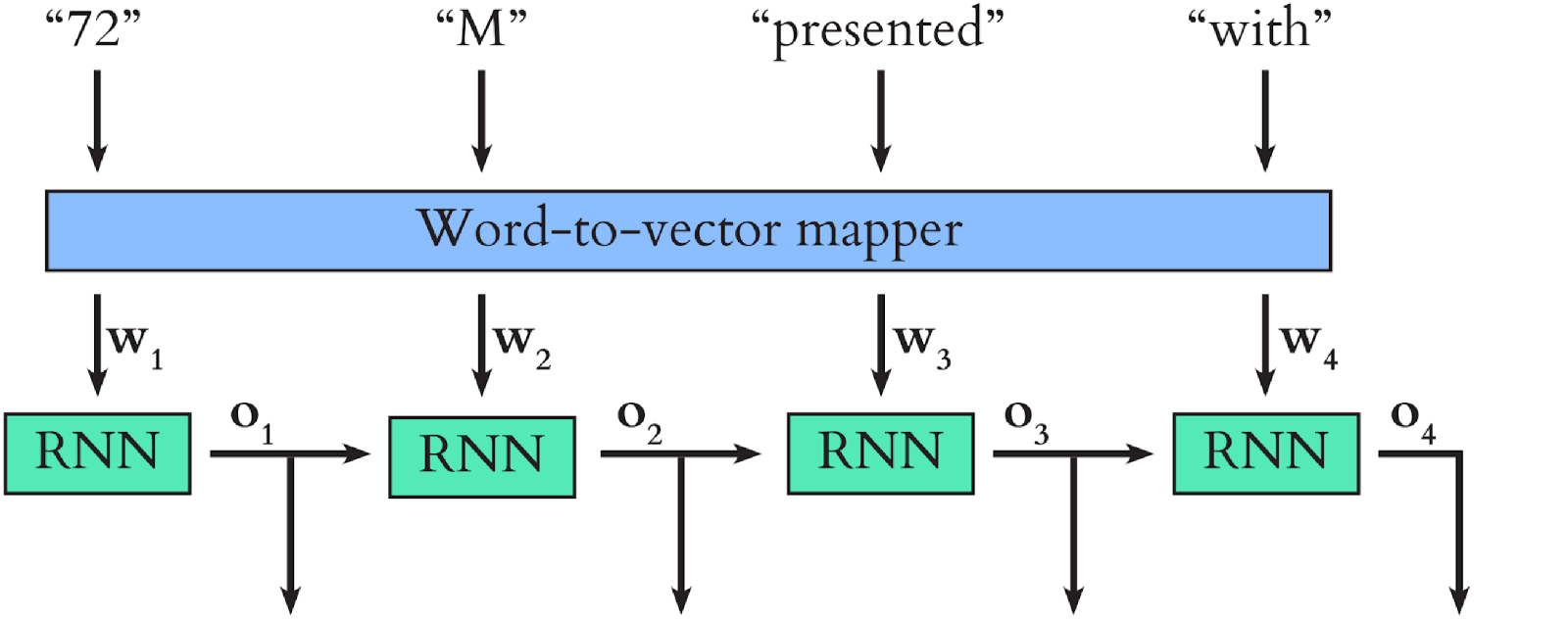
We trained our model using text notes and clinical codes from the MIMIC dataset. The text in question was the discharge summary for each patient, which summarises their stay in hospital. The full discharge summaries in MIMIC contain explicit lists of patients' diagnoses, but we wanted to train a model to predict clinical codes from overall context, rather than simply extracting this diagnosis list. To achieve this, we heavily abridged the discharge summaries, so that each contained just the patient's 'history of presenting illness'.
Problem 2: Most diseases are rare
During training, the model learns to represent each clinical code as a vector. When a new discharge summary is input to the trained model, it represents the text as the final RNN output, then uses this output and each code vector to calculate the probability that this code applies to the patient in question. The distribution of 'primary diagnosis' clinical codes in the dataset (over 55000 patients) is shown below.
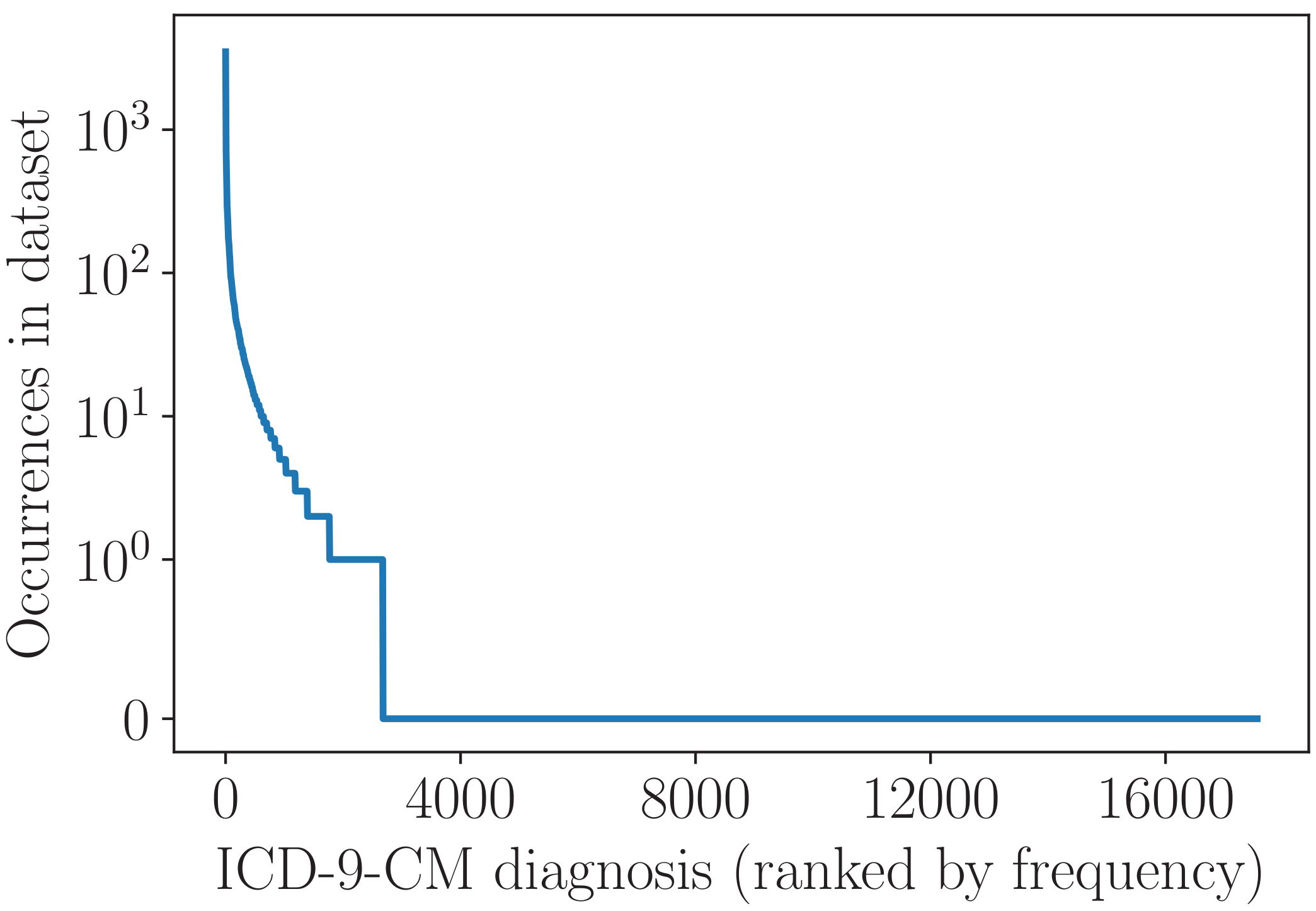
We can see that, whilst a few diagnoses occur very commonly, the vast majority occur rarely or not at all. One simple way to learn the clinical code representations is to treat each diagnosis in isolation, i.e. to learn about each one from just the patients in the dataset who have that diagnosis. This works fine for common diagnoses that occur hundreds or thousands of times, but many of the rare diseases simply don't appear enough for this approach to be effective.
Recall that clinical codes are organised in a tree-like hierarchy? We used this to our advantage by learning a representation of each node in the hierarchy (the roots and branches of the tree) during model training, and composing the representation of each diagnosis (the leaves of the tree) from these. This way, we share information between diagnoses, because they have common ancestor nodes. For example, when we encounter a patient with asthma we can learn something from them about respiratory disease in general, and use this to help us diagnose e.g. COPD. The diagram below shows how this process works for our patient with pnuemonia.
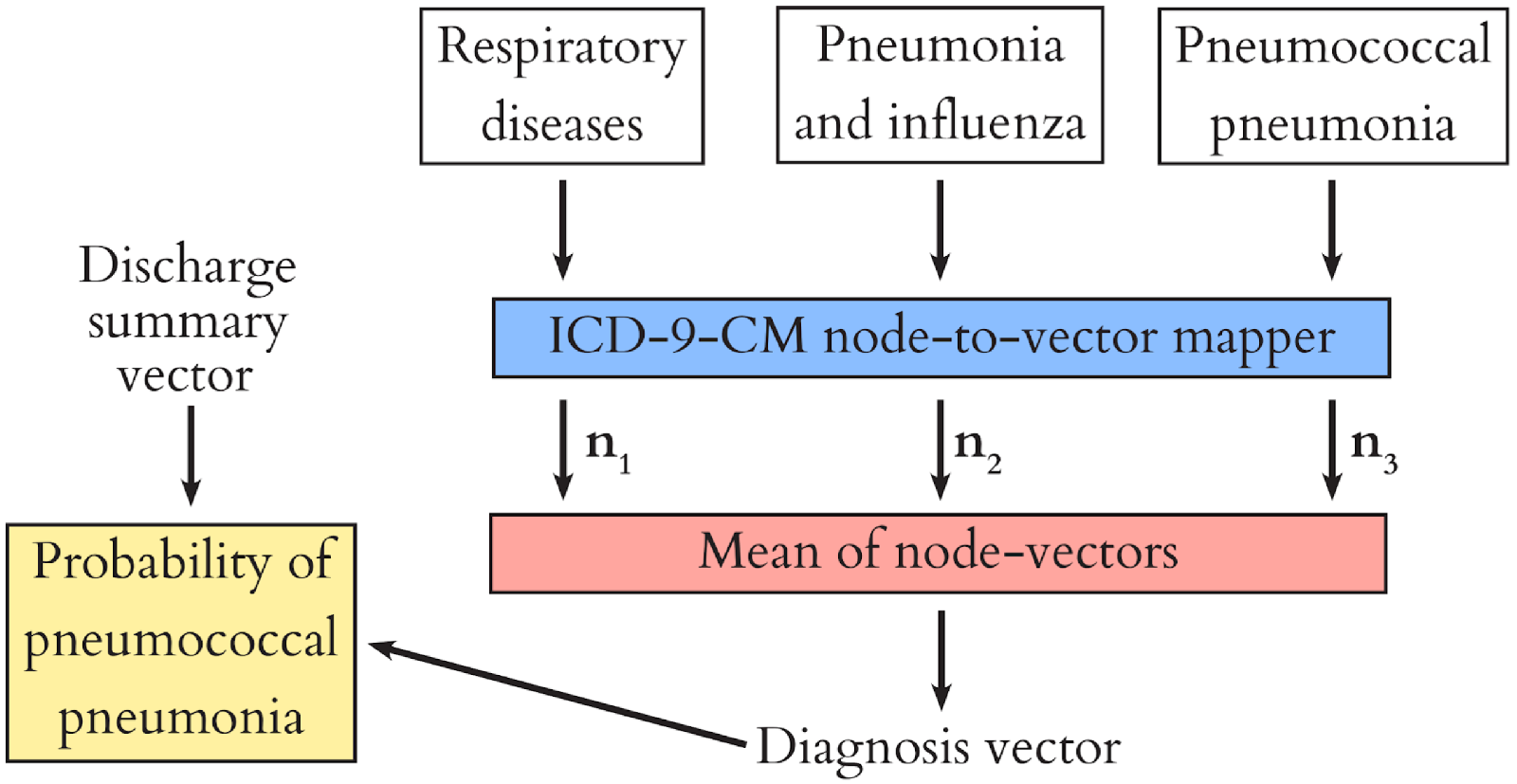
The paper itself contains much more detail and discussion of this project. If this post has piqued your interest, please take a look and get in touch via Twitter with any questions or comments.